The benefits of hyperpersonalization are clear: better engagement, higher conversion rates, increased loyalty, enhanced brand appreciation, and more. Of course, we could dive deeper here, exploring examples, KPIs, best practices, and so on. However, our focus in this paper is to shed light on another critical aspect: the data foundation required to enable and enhance hyperpersonalized interactions.
After all, to personalize communication effectively, you need to know your consumer. Do you understand your database? Is your information organized and ready for use? Are you utilizing the right tools and technologies to improve predictions? In essence, are you prepared for the next stage of interaction?
In this paper, we will outline the key steps to the path for hyperpersonalization, and provide a brief self-assessment at the end. Be honest—are you truly ready? Let’s explore this journey together.
The Hidden Necessity: Data as the Foundation of Hyperpersonalization
It might seem obvious that personalized communication with your customer requires a deep understanding of who they are. Yet, many companies struggle at this fundamental step. Some marketers are eager to dive into personalization without fully appreciating the importance of truly knowing their customers. This isn’t just about understanding the general behaviors and interests of the average consumer. When we talk about "knowing" your customer, we mean something far more comprehensive:
“You must dive deeper, gathering and analyzing data on each unique individual, predicting their next move, and anticipating their needs with precision—all while respecting ethical and legal boundaries.”
Reaching this level of understanding is essential for true hyperpersonalization. Only when you can anticipate your customers' needs in an automated, data-driven way—constantly refining your predictions—can you begin to deliver hyperpersonalized communication that truly resonates. Achieving this requires a structured approach, which we'll explore in the following four key steps.
What is a CDP?
A Customer Data Platform (CDP) is a centralized system that collects, integrates, and manages customer data from various sources. It provides a unified, comprehensive view of each customer, which is essential for effective personalization. Some companies offer CDPs as a packaged service, promising a turnkey solution for managing and utilizing customer data. However, in this paper, we explore the underlying structure and processes required to build a platform that supports your specific business needs. By understanding the architecture behind a CDP, you can tailor the system to your unique requirements, ensuring that your efforts in hyperpersonalization are built on a solid foundation.
Path for preparing data to hyperpersonalization
1. DATA Gathering
The first step toward achieving hyperpersonalization is gathering as much relevant data as possible. This data collection should include a wide range of customer information, such as transactional history, browsing behaviours, preferences, and interactions across various channels. While collecting this data, it is essential to adhere to privacy regulations like GDPR, ensuring that customer data is obtained with explicit consent and handled with the utmost care. Transparency in data practices not only helps in building trust with your customers but also fosters long-term loyalty. Prioritizing the collection of first-party data—information directly gathered from customer interactions—ensures accuracy and relevance, laying a strong foundation for personalized experiences.
To gather data effectively while respecting privacy, businesses can use first-party trackers and other compliant tools. Many systems nowadays collect important information about customers, such as CRM platforms, social media monitoring tools, e-commerce systems, and even internal controlling system. All the data generated by them can be seamlessly integrated via APIs (Application Programming Interfaces) that allow the extraction and consolidation of the data into a single repository, such as a data lake. - A data lake is a scalable storage system that holds raw data in its native format until needed - This integration allows businesses to centralize all customer-related data, regardless of its source, into one unified location. This is the first step for creating a comprehensive view of each customer, making it easier to analyse interactions, behaviours, and preferences.
An essential aspect to consider while gathering information is adherence to privacy laws. You can consult the European Data Protection Board or various resources online about GDPR. Alternatively, feel free to reach out to us for support in understanding how to gather as much information as possible without any risk.
Extra Content:
First vs Third party data
First-party data is information collected directly by a company from its customers or users through
interactions with the brand, such as purchases, website visits, or social media engagement. This data is highly valuable because it is unique to the brand, offering insights into consumer behavior that can be used to tailor marketing strategies and enhance customer relationships. Compared to third-party data, which is collected by external organizations not directly involved in the customer’s interaction with the brand, first-party data is considered more reliable and compliant with privacy regulations like GDPR, provided that it is gathered transparently and with the user's consent.
Due to ethical concerns, regulatory pressures, and the superior quality of first-party data, brands are increasingly encouraged to rely on this data and use their own tracking tools to continue gathering valuable consumer insights in a compliant manner.
2. Data Organization and Cleaning
During the data-gathering phase, companies often rely on various APIs or specialized tools to connect and consolidate data from multiple sources into a central repository known as a data lake. This centralized approach allows organizations to aggregate diverse data types, including structured, semi-structured, and unstructured data, making it easier to manage and analyse.
However, data, in its raw form, is merely a collection of facts and figures. Without proper organization and accessibility, even the most comprehensive datasets hold little value. The true power of data lies in its ability to generate actionable insights that can drive timely and informed decisions. For this to happen, data must be structured in a way that allows it to be easily accessed, analysed, and utilized effectively across an organization.
Once data is collected in the data lake, it must go through normalization. The first step in employing the potential of data is preparation. This involves cleaning the data to remove biases, correct errors, and standardize it for use. Clean data is the foundation of accurate analysis; even minor inconsistencies can lead to misleading insights, which in turn can result in flawed business strategies. Additionally, eliminating bias in data is crucial, as it ensures that the resulting analysis reflects reality, not distorted or skewed interpretations.
Finally, the organized and cleaned data must be prepared for visualization and analysis, which we will cover in the next step
3.Data analysis and insight generation
Once data is organized and cleaned, the next critical step is to ensure that it is easily accessible to stakeholders who need it for insights and analysis. This is where visualization tools come into play. There are many tools available in the market, with Power BI and Tableau being among the most widely used. Regardless of the tool chosen, the objective remains the same: to make data easy and quick to access, enabling users to derive meaningful insights without unnecessary delays.
Data visualization is not just about presenting numbers; it's about telling a story with data. Visualizations can range from simple KPI dashboards, which provide an at-a-glance view of key metrics, to more complex analyses that allow users to explore data relationships and trends. These graphical representations are crucial for ensuring a clean and clear understanding of the data. By making the data visually appealing and easy to comprehend, stakeholders can quickly identify patterns, trends, and anomalies that might otherwise go unnoticed.
In addition to standard KPI dashboards, more advanced visualizations and cross-analyses can be conducted to dig deeper into the data. For example, cross-analysis might involve comparing different datasets to identify correlations or trends that can inform strategic decisions. This could include analysing customer behaviour across different segments to identify opportunities for targeted marketing or exploring sales data in conjunction with market trends to forecast future performance.
These tools and analyses empower decision-makers across the organization by providing them with the insights they need to act confidently and effectively. The goal is to transform raw data into actionable intelligence, enabling stakeholders to make data-driven decisions that can improve business outcomes.
4. Predictive Analysis and Automated Interactions
The next step in preparing your data for hyperpersonalization involves anticipating customer behaviours, preferences, and future trends based on historical data—this is known as predictive analysis. By leveraging advanced algorithms and machine learning models, you can forecast what customers are likely to do next, such as making a purchase, engaging with a promotion, or even considering a competitor. These predictive insights are invaluable for crafting proactive strategies that align with customer needs, thereby enhancing satisfaction and loyalty.
The accuracy of these predictions depends on the quality and quantity of the data, as well as the sophistication of the algorithms used. This is why each step in data preparation is crucial—clean, organized, and well-structured data forms the backbone of reliable predictive models.
Once predictive analysis provides valuable insights, the next step is to connect them with automation rules and CRM tools. By integrating predictive analytics with CRM systems, businesses can automate customer interactions, ensuring that each customer receives personalized communication at the right time with the right content.
This combination of predictive analytics and automation creates the "magic" of hyperpersonalization. Not only are communications tailored to the individual, but they are also delivered in a timely manner, often anticipating the customer's needs before they even realize them. This level of personalization fosters a deeper connection with the customer, as they feel understood and valued by the brand.
How far are you from hyperpersonalization?
As we've explored, the journey to hyperpersonalization involves more than just implementing the latest technology or tools. It requires a robust data foundation, meticulous preparation, and a deep understanding of your customers. From gathering and organizing data to analyzing it for insights and leveraging predictive analytics, each step is crucial in building a personalized experience that resonates with your audience.
Before diving into hyperpersonalization, take a moment to assess your readiness. Do you have the necessary data infrastructure in place? Are your data collection and management practices aligned with privacy regulations? Are you equipped with the tools and expertise to analyse and act on data insights effectively?
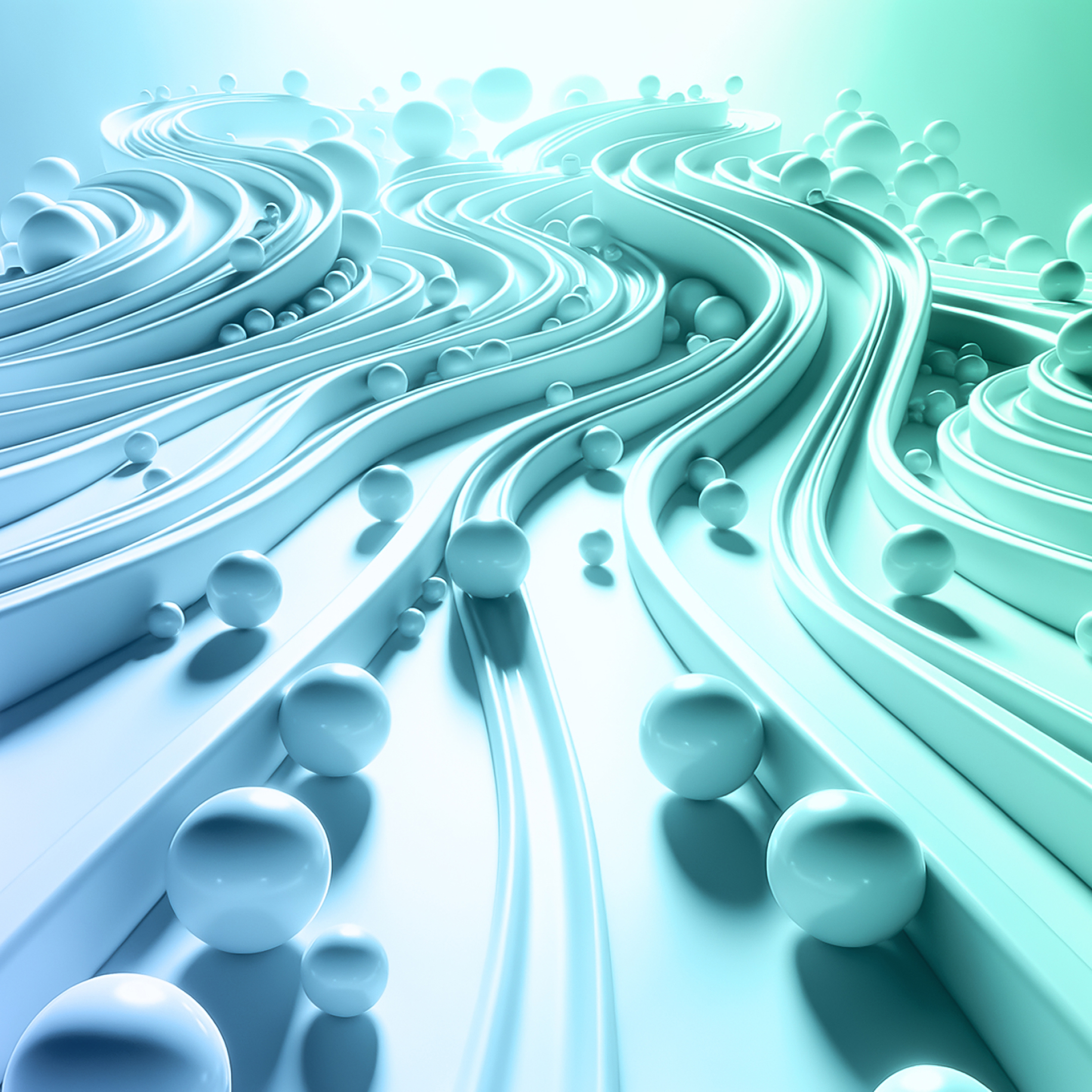
Take our self-assessment test now and download the whitepaper here!